5 Key Characteristics of Data Quality for Asset Management
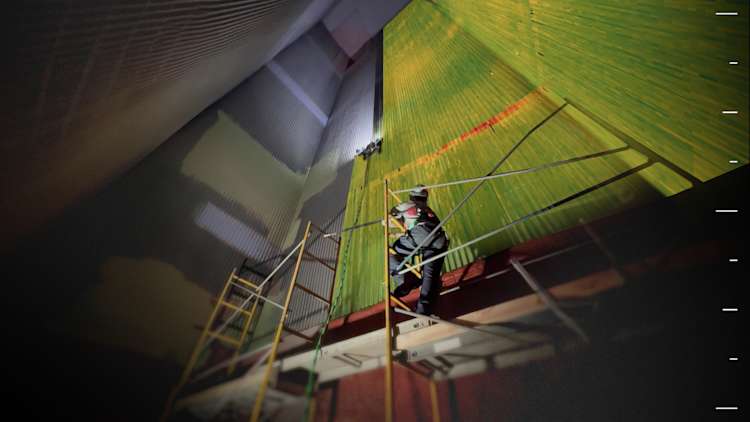
As mathematician Clive Humby famously stated, “Data is the new oil.” Like oil, we need to refine and process our data to make it useful – but this concept misses a foundational first step. Before analysis begins or artificial intelligence (AI) algorithms run, it’s critical that you first ensure the quality of the “oil.”
An often overlooked aspect of AI is that algorithms cannot differentiate between good and bad data. Instead, it works on logic, learning from patterns in the provided data. Robust, high-quality data sets are critical for training and validation, ensuring that AI models are accurate and reliable. If data is insufficient, analyses and algorithms could lead you down the wrong path.
Understanding where your raw data comes from is essential to knowing whether the outputs will be valuable, trustworthy, reliable, and actionable.
A Big Data Quality Conundrum
In a recent study, 89% of executives stated that a high level of data quality was critical for the success of their organizations, yet 75% indicated that they don’t trust their data. This poses quite a contradiction.
As companies strive to accelerate decision-making processes to keep up with increasing demands, they often only do so at the sacrifice of quality. Without the right methods and technologies in place, decision-makers are left to plan their next steps using data riddled with assumptions, biased inputs, incomplete information, and inaccuracies.
Insufficient data in the industrial and defense sectors results in extensive time, budgets, and resources spent on the wrong objectives. Not only can poor-quality data harm your bottom line, but it can also be hazardous, with sites experiencing unexpected equipment failures and catastrophic events.
As many people say: garbage in, garbage out. While this concept is easy to understand, there is little focus on verifying the data that fuels our strategies, processes, data systems, and action plans. Even the most sophisticated data analytics platforms and AI algorithms will fall short if fed poor-quality data.
What Makes Good Quality Data for Industrial Asset Management?
The more you understand about an asset’s health, the better you can operate and maintain it, now and in the future. Maintaining equipment with a data-driven approach enables facilities to reap significant benefits, including reduced downtime, faster root cause analysis, improved return on invested capital (ROIC) and internal rate of return (IRR), informed capital expenditure (CapEx) planning, and extended useful life of critical assets with scalable standard operating procedures (SOPs) across entire fleets.
However, not all data is created equal. Quality data must have these five traits:
-
Accuracy: Is your data correct? Is there a marginal error?
-
Completeness: Is your data comprehensive? Does it provide full coverage?
-
Precision: Is your data high density?
-
Reliability: Is your data source trustworthy and consistent?
-
Timeliness: Is your data accessible in an actionable timeframe?
If any of these traits are missing, the data output becomes unusable. Many traditional nondestructive testing (NDT) methods that have been relied upon for decades leave most of these boxes unchecked.
For example, visual and manual data collection methods are subjective to the inspector's skill level, documentation, and interpretation. Visual inspections only capture surface-level damage visible to the eye, and manual inspections take readings in specific yet limited and dispersed areas of an asset, leaving large portions completely unassessed.
Traditional inspection methods usually require scaffolding or man-lifts for inspectors to access various points. Securing and implementing additional resources adds significant time to the process, further delaying analysis and maintenance execution. When it comes to industrial asset management – added time can mean added risks. These outdated methods don’t cut it, especially with so much riding on the line.
How to Obtain Good Quality Data
Effective industrial asset management comes down to the data. You shouldn’t rely on yesterday’s archaic data collection and analysis methods to ensure that assets can meet today's and tomorrow's demands.
Alternatively, asset owners and operators can leverage advanced automated technologies to ensure accurate, complete, precise, reliable, and timely data collection at speeds and densities unmatched by conventional methods.
For example, Rapid Ultrasonic Gridding (RUG) is an automated, robot-enabled ultrasonic testing (UT) method that replaces traditional manual gridding. The robot continuously captures data as it moves, collecting 1,000x more data at speeds 10x faster than traditional methods. The robot has built-in encoders for localization that enhance accuracy and repeatability as data is captured.
The raw data collected by the robot is imported into a data analytics software platform to create a full-coverage, high-quality digital record. The platform integrates this data layer with historical maintenance records, operating conditions, OEM specifications, and other relevant data sources in one centralized location to generate actionable insights through fully comprehensive and accurate maps, models, digital twins, and prioritized repair plans.
By pairing the high-fidelity data collected by the robots with historical information, the analytics platform can get a more accurate baseline for how assets are aging over time. In-depth, high-quality data forms the foundation for an asset management tool that provides accurate, valuable insights.
Build a Facility of the Future with Predictive Maintenance and AI
Today’s advances in automated data-collection robots, fixed sensors, and data analysis software provide an opportunity to access and analyze data in ways that weren’t previously possible. The sheer quantity and depth of data points make advancements like AI possible.
Predictive maintenance driven by advanced analytics and AI can accelerate decision making, remove guesswork, and reduce operational downtime. You can predict what will fail and automate repair plans using AI to maximize budgets and increase useful life.
Data quality has never been more important, especially as we increasingly turn to software platforms and AI systems to guide our decisions and next steps. You can completely transform how you monitor and maintain critical assets, but only if you ensure your “oil” – or data – is accurate, complete, precise, reliable, and timely. Quality data ensures you prioritize work based on what matters, saving time and money while minimizing failures.
The right technologies will unlock quality data to fuel analysis and drive positive outcomes. Facilities of the future gain unmatched visibility into today's and tomorrow’s issues by leveraging the most advanced, connected, and intelligent technologies.
Analytics tools with a strong data foundation will help close the loop between strategy and maintenance execution – empowering teams to understand, collaborate, and confidently make strategic decisions that ensure asset networks are reliable today and future-proofed for tomorrow.